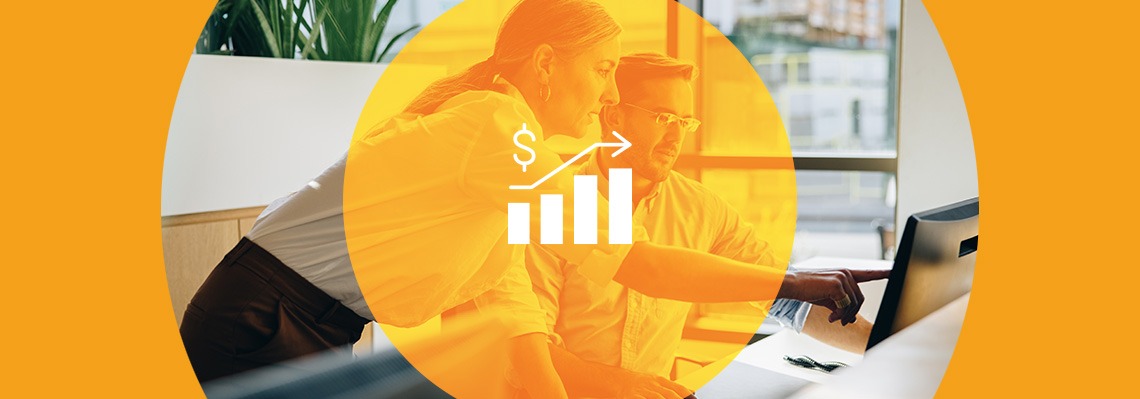
Four Ways Your Biased Media Mix Model Could Lead You Astray
Media Mix Models are becoming more important as privacy slowly erodes tracking capabilities — which is decreasing measurability through technological means. Media Mix Models (MMM) try to calculate the sensitivity of your revenue (or other KPI) to your media channel spends. It does this by building a statistical model of how we know marketing works (spend in channels will have a non-negative impact on your business — in nearly all cases) and by using data of your spend, other factors of your business, and your KPI to calculate the sensitivities between the factors of the business and the business that best reflects the actual observed data.
This is similar to any standard causal statistical model — it seems fairly straightforward, but there are four major ways in which your MMM can be biased. And these biases are a big deal because a biased model will still generate a logical output, but it could be significantly inaccurate, which may be worse than no model at all.
Here are the four biases to watch out for:
Aggregation Bias
- If you do not collect data at the appropriate level of granularity, you may be subjecting yourself to Bias of Aggregation. The classic example in MMMs is when you have a business across a country, but there are different regional effects (think the US and DMAs) that make your business slightly different from region to region. If you built your MMM at the national level, you could be calculating those sensitivities from media to your business that are biased because each DMA acts differently — and when all DMAs act (even slightly) differently, this can add up to a drastically incorrect MMM.
- The solution is to model at the DMA level using a hierarchical technique that takes advantage of the information difference between the DMAs and the information similarity across DMAs.
Variance and Collider Bias
- Sometimes, a media channel may have no variance (no change in the level of spend over time), or certain media channels may be highly correlated and include two or more highly correlated variables that will bias the coefficients (sensitivities). This is a well-known problem, and there are many ways to deal with this such as aggregating variables, dropping variables, etc.
- Lesser known in this realm is the Collider bias, which is including a variable in the model that is caused *by* both an independent variable and your KPI — sometimes in an MMM, this could be app usage (which may be driven by media spend and by the KPI).
Missing Variable Bias
- Making sure you include all potential influencers of your business in a MMM is critical — if you end up missing one big driver of your business, all other estimates are still technically “correct” but are very misleading. Once you include that missing variable, you’ll often see the sensitivities shift dramatically for the other channels.
Rogue Optimization Bias
- Models are conceptually very simple: Set up the relationship between your media channels and other influences of the business on the KPI, feed some data in, and let the model find the relationship strengths. Conceptually, this is accurate, but in reality, this “relationship strength finding” of the model is not so simple. Models will find the “optimal” relationships between your media channels and your KPI, but the models don’t know how the world really works — so the data scientist must help guide the models for basic things like each DMA may respond to channels differently, media impact on KPI should virtually never be negative, media impact on KPI is never going to be 1,000,000 to 1. All of these things need to be encoded into a model to ensure results are both mathematically accurate and reflect reality. The most proven way to do this is by using Bayesian methods that utilize a prior.
MMMs are phenomenal tools; they help diagnose which channels are working and they guide important investment decisions, especially when there are no other methods available. As MMMs become more common, however, it’s very important that marketers discern the quality of the partner and the model output when implementing them.